Efficiency and speed are crucial for any company aiming to lead the market, and this is especially true in R&D, where leaders are under increasing pressure to reduce product development cycle times. R&D is an expedition into the unknown, requiring a mix of scientific techniques and perseverance. Dead ends and setbacks are not just common but expected. These challenges can obstruct your quest to be lean and first-to-market, especially if experiments are not performed as efficiently as possible.
So how do we turn this R&D experiment process….
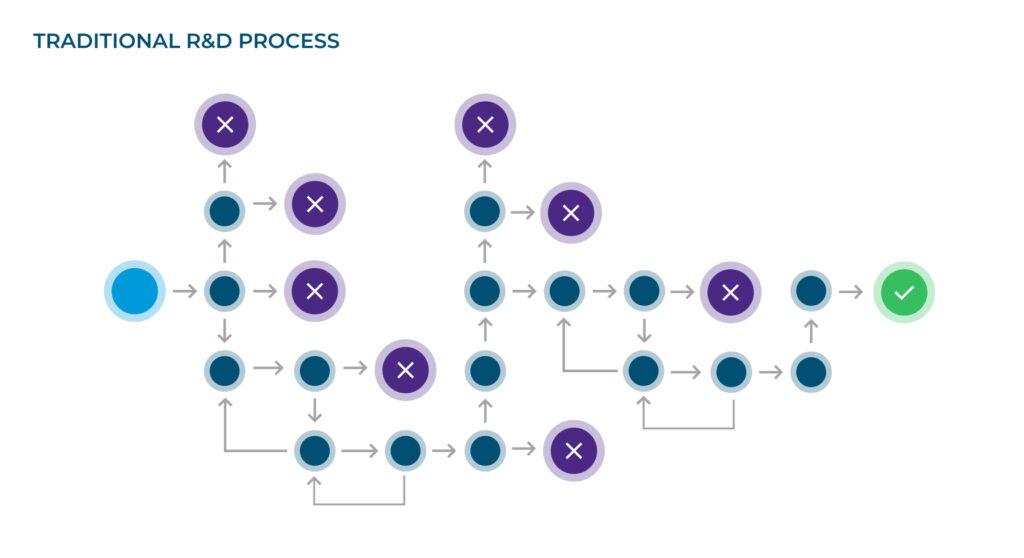
…into this experiment process?
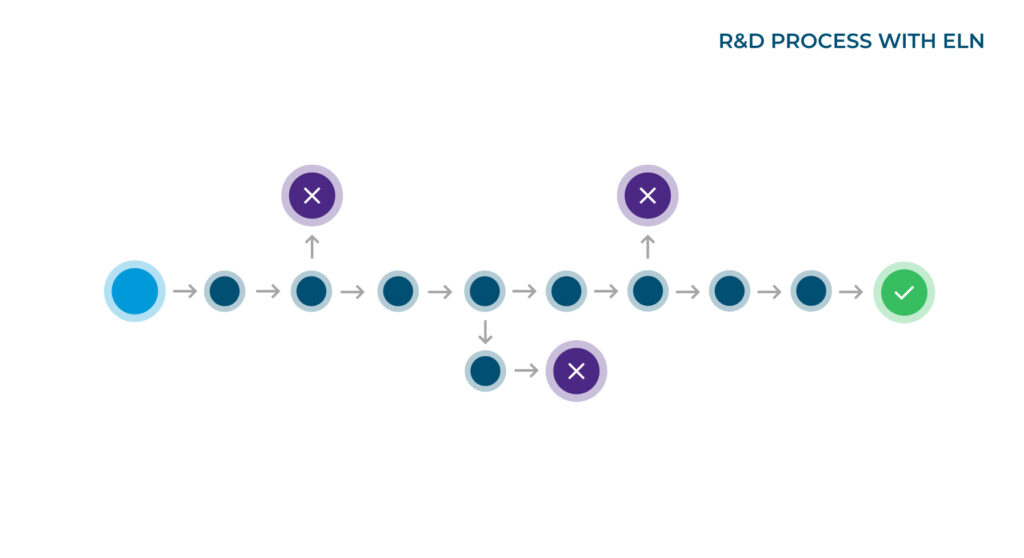
Currently, it’s not uncommon for scientists to explore many different ideas to achieve a result or even spend months just trying to reproduce past results. By minimizing the number of loops and wrong turns – whether caused by failed replications, human error, or just intrinsic uncertainty – the savings in time and resources can be significant. A commitment to laboratory data collection and high data quality can improve an R&D lab’s ability both to reproduce results more easily and take a more direct path to the desired outcome.
Data Quality: The Foundation of Innovation
We believe the core of scientific innovation lies in robust data. Data-derived insights help shape decisions and drive research. High-quality data is not just a tool for decision-making—it’s a strategic asset that can accelerate and expand an R&D organization’s capacity to generate intellectual property (IP). Yet, with the rapid increase in data volumes, types, and silos, many R&D labs struggle to establish effective systems for laboratory data collection as well as managing, sharing, and mining insights from crucial information.
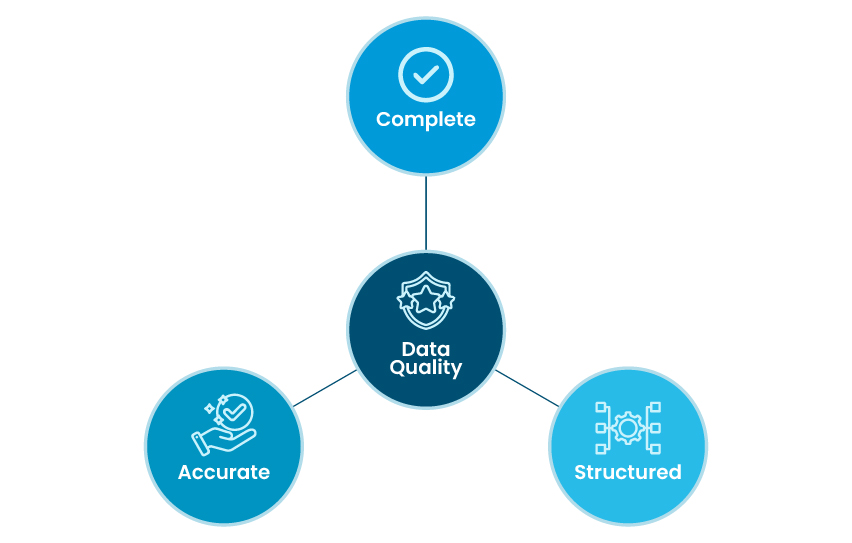
The Role of Data Quality in R&D
“Data quality” is an overarching term that encompasses the accuracy, completeness, and structure of data. Correct, clean, and easily accessible data supports full experiment traceability – a critical step in achieving compliance and generating IP. By committing to data quality, R&D teams can improve reproducibility, collaborate more effectively, and maximize the value of their data. While data accuracy is self-explanatory, this blog will cover how to achieve data completeness and how structured data helps to propel innovation.
Achieving Data Completeness for R&D Experiments
Data completeness encompasses any detail that could conceivably affect the outcome of an experiment. If we look back through history, the documentation of methods, calculations, and data is pretty much the defining feature of science. If an experiment is not reproducible during the early stage R&D phase, then it can’t be practically applied and commercialized. The more detailed the records and documentation are, the easier it is to reproduce. Having complete data improves reproducibility rates and minimizes the number of experiments needed to achieve a breakthrough.
Essential Data for R&D Laboratory Data Collection:
A comprehensive experiment record should contain data such as:
- Precise procedural steps, their sequence, and details about who performed what, when, and where
- Details on materials used, including container, lot number, and history
- Exact quantities used
- Any controlled variables like temperature and time
- Uncontrolled variables, such as lab ambient conditions
- Equipment used and its settings, including complete calibration and service histories
- Analytical methods from raw data to results
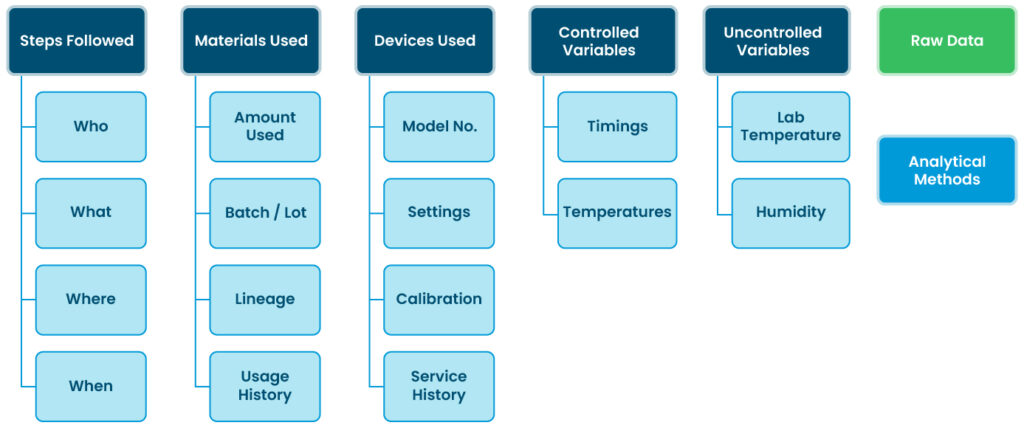
Despite its importance, most R&D documentation still occurs on paper. Manual record-keeping by researchers is labor-intensive, and further complicating matters, researchers frequently write up their documentation weeks after conducting experiments, which can also impact the accuracy of the record if details are misremembered.
Data Structure: Unlocking the True Value of your R&D Data
Data that is isolated and disconnected in a series of paper lab notebooks is of little value. When data is centralized and structured in a secure and encrypted digital platform, it becomes accessible, traceable, and auditable. This supports both compliance efforts and IP protection.
Centralizing research in a digital environment also empowers global, interdisciplinary collaboration, which is essential to today’s interconnected landscape. Sharing a common R&D platform allows teams to establish communication channels, foster knowledge exchange, and optimize team workflows to eliminate unnecessary steps, reduce bottlenecks, and achieve maximum productivity.
Structuring your data in a digital environment also enables your data to be fed into statistical or machine learning models. These types of models can then find patterns, predict outcomes, and generate insights that would be impossible to discern manually, helping your organization reduce redundant experiments and get to market faster. This ultimately enables your organization to build upon scientific research, helping drive the development of new products and innovations.
The impact of data quality on R&D efficiency cannot be overstated. Accuracy, completeness, and structure each play a pivotal role in optimizing the experimental processes. To maximize data quality, R&D labs need to be equipped with modern digital tools that automate data capture and analysis. R&D platforms like an Electronic Laboratory Notebook (ELN) offer a promising path forward for scientists by providing centralized control, automation capabilities, and interconnectivity with lab equipment, devices, and other systems.
Integrating a tool such as an ELN into their daily workflows allows scientists to capture information effortlessly during experiments. This streamlined approach not only alleviates the documentation burden, but also accelerates discovery and helps deliver innovations to market faster.
A version of this article was originally published in R&D World.